I recently ran a workshop with Dr Christine Couper from the University of Greenwich and HESPA around how planners are making use of the data being collated for learning analytics. The workshop started with presentations to set the context and give some examples of how this data is already being used.
Christine talked about how they are using data for learning analytics and starting to look at curriculum analytics. I set out how Jisc is looking to expand the sources of data in the analytics service to include more learning activity data sources, intelligent campus data, curriculum data, employability data and also looking at student success, health and well-being.
Kevin Mayles form the Open University described their learning analytics jourmey and how they have been engaging academics in teaching data. James Hodgkins, University of Gloucestershire talked about their implementation of learning analytics and library data and measuring module quality. Lee Baylis from Jisc talked about the outputs from recent analytics labs and the latest invitation for participants. Finally Niall Sclater described the different strategic approaches and drivers for institutions implementing learning analytics.
How planners want to use the data
Fuelled by all these ideas we then asked the participants to suggest how they would like to be able to use this data. We did this by getting then to write some user stories which we then categorised.
- Using data to improve support students and measuring effectiveness of interventions
- Curriculum analytics looking at module success and patterns of module design
- Improving recruitment and progression, widening access and getting better measures relating to demographic groups of students.
- Improving data quality and joining data sets
- Gathering data around how students are feeling, emotional and sentiment analysis to both support students and get a better measure of student satisfaction
- Early entry analytics, identifying the data points that can be most informative to success.
- Developing a generic high level strategy board maybe using some common metrics
- Identify the attainment gaps in student cohorts
- Being able to predict the outcome or grade for a student
We then worked in pairs to explore some of these categories further, looking at the challenges that would need to be overcome and the data required.
The groups chose
1. How to make effective interventions?
This built on the existing idea of using learning analytics data to identify at risk students and make timely interventions. The main challenge was seen as the quality of the data for both deciding when to make an intervention and recording the intervention. A reliable data set will need to be maintained to be able to make meaningful and consistent interventions. When it comes to making and recording interventions there is a challenge in deciding at what point to make an intervention based on the data and information to hand. Who will be accountable and responsible for making sure an intervention happens and the student is supported. Making this happen may require new processes to support interventions or at least to integrate it with existing processes. There could be ethical concerns if this data was used for staff performance management and monitoring. It would be essential to make sure the students are aware of how their data is being used.
Some of the data required would be
- Sufficient data on all students to be able to decide when to intervene
- Data around the interventions and indications of personal circumstances or reasons for withdrawal to be able to model effectiveness of the intervention process
- Contact data to be able make an intervention
- Qualitative data and feedback from staff and students
2. What makes a course successful?
The idea here is to look at existing successful courses and identify what made them work and use that to make other courses equally successful or create new courses. To quote “Try to define the unknown based on what you have or a developing a new course based on existing modules.”
The group suggested looking at combining the competition/market information with internal factors to determine what might be successful. Others measures of success could be entry stats, retention, employability, students satisfaction and quality of the data. Mining this information could be a challenge as not all of the data is readily available.
3. Reporting data around widening participation
Learning analytics data offers the opportunity to provide better insights into the performance and success of students in widening participation groups. There are challenges around identifying hat data is available and accessing the data we don’t have. There are also costs involved in getting his data. Joining up data sets to make them cohesive can be tricky if no common identifiers are available. A drawing of the data to be collated into one store to be analysed was produced by the team.

4. What can student society membership tell us?
The idea is to see there is an impact of engaging with clubs and societies on a student’s learning journey. This could be collated with learning analytics data to see if good students also engage with clubs and societies. It could also be combined with data on student well-being to see if there is any relationship.
The challenges would be around accessing the student’s engagement data for societies and the willingness of the students union to share this data, assuming they collect it and it ids reasonably complete. The students will need to be happy about being tracked on society membership and attendance.
A schema for societies data and standards for its collection would be required which could include type classification, price of events and membership, frequency of events and calendar of events.

5. Can we predict a students outcome?
The ideas here is to build a model to help students see what grade they will achieve if they continue on current pathway or could achieve if they performed better. This could be combined with a tool to recommend module selections that would maximise success. There are always challenges in being able to predict final grades and many factors to be considered. It would also be useful to be able to suggest what behaviour(s) you could nudge to improve outcomes for an individual student.
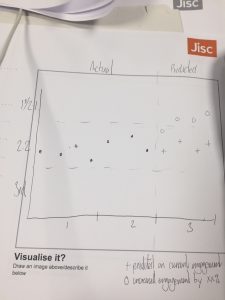
It was interesting to see what ideas for using learning analytics data planners would come up with in a short activity. If you are interested in learning more about the latest Jisc analytics labs take a look at the blog.